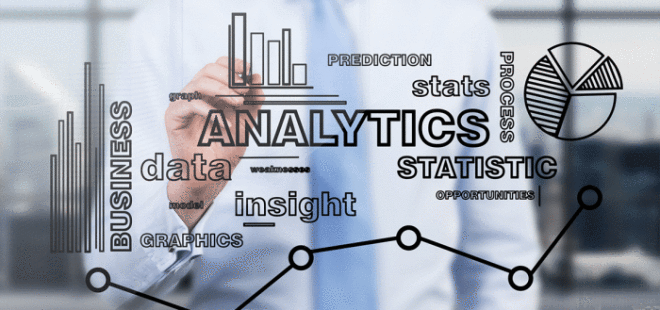
A 2016 Business Insider and World Economic forum report ranked Uganda as the most entrepreneurial country on the face of the earth. The same report however, noticed a very high mortality rate for Ugandan businesses before celebrating their first birthdays. This is not surprising to us, entrepreneurs and development practitioners, because the general perception of (would-be) business owners is that a successful business is a product of constant trial and error process, rather than a careful scientific process of planning, measuring and pivoting a business model.
For a small business operating in thin markets such as Uganda, characterized with poor support systems (infrastructure, finance, talent and regulations), careful planning is even more important because it can forecast the chance of profitability (at planning stage), measure the performance of a given model and tells you what/when to pivot. More than ever before, businesses in such markets need to realize that data has value.
To further highlight this, we present the true case of AgroMech (U) Limited (not real name), a mechanization service provision company in Northern Uganda that offers a whole range of farm mechanization services (see box). This case points to the fact that there is a voluminous amount of data (ranging from financial to non-financial; quantitative and qualitative) involved in running a business. It also demonstrates that it is important to empower a business like AgroMech Limited to always integrate data when making business decisions. With only a basic understanding of the importance of the data, it is possible to win in a marketplace more than lose, and attract more success to a business. We peg our argument on evidence which indicates that companies that engaged in data-driven decision-making experience a 6% increase in the productivity and the output of the company over the companies that do not[1].
Our Motivation
From the development practitioner’s point of view, the motivation for the data collection task and usage is one that is integral to everything a development program should be doing. Like Dr. Ben Taylor said, we need to know what we don’t know, what we can and should know. As development practitioners aiming to stimulate systemic change, it becomes imperative to know what can be done to stimulate the much-desired change. All along we understood the cost involved in mechanization[2]and so we wanted to know how the mechanization actors could be made profitable.
AgroMech received support to design a financial model which measures current performance and projects future financial situations. Even though the model projected profitability using a sketchy set of historical data, the company managers were however not sure how to actually turn profits in the turbulent mechanization market system of Northern Uganda. After all, the company’s bank balances had never reflected such positive positions during that historical period. The company was worried and almost deciding to quit, but saw a fresh opportunity to achieve competitiveness and profitability using data driven decision making. But first, AgroMech had to build an infrastructure (simple system of collecting and storing primary transaction records and a computer to run the excel sheets containing the models) and a culture of handling its financial data with discipline, as well as develop a behavior of data-driven decision management.
The challenge was that, in a thin market like that of Northern Uganda, offering a new value such as mechanization services to smallholder farmers means money practically often moves in and out of the business without going through the banking system or even without reaching the physical business premises. Ultimately, the business had a responsibility to keep data (inform of transaction records), after which they received support to analyze and make sense of (again, using simple excel models). The outputs are used to drive the decisions by the business with development practitioners only taking a role in advising the business.
Quantitative Data for decision
AgroMech tracked financial information for a period of two years from which the financial statements (income statement, balance sheet and cash flow statement) were produced for each year. A financial model was developed to generate a summary of the company’s performance based on certain variables such as cash flow projections, depreciation schedules for equipment and implements, debt service, receivable days, rate of inflation, etc. over monthly, quarterly or annual periods. The model also projected the same variables over 3 years (2019-2021), and provided capabilities to test various outcomes after change in one variable or another. This capability particularly allows the AgroMech Limited to really quantify the implications of each business decision it makes, policy it puts into place, the financial obligations it agrees to, and the conditions imposed upon it by other partners such as lenders and/or investors if any. The business now has data that can predict its future state based on various growth scenarios. The management’s role now is to translate the set of scenarios with best outcomes to performance reality for the company. Quantitative data collection and records management is no longer seen as a tedious unnecessary task but rather as a culture that the business cannot survive without.
Further, AgroMech now uses ratio analysis (liquidity ratios, profitability ratios,turnover ratios and debt ratios) that the model generates to determine the financial health and operational efficiency of the company at any given time. Management utilizes it to compare performance of various mechanization services to determine which one to grow and which one to systematically phase out. These data not only now drive business decisions, but the company is getting ready for an investor pitch while leveraging on these data with a renewed set of confidence. The company is seeking private equity funding and/or angel investors with a solid data-driven understanding of its offer and payback possibilities.
Non-numerical data for decision
Through mechanization clinics, a business practice where farmers interface with equipment and farm machinery; AgroMech Limited also collects a number of numerical and non-numerical data. The numerical data include number of farmers, demand,aggregated acreage etc. Non-numerical data include response patterns, farmer readiness for mechanization, distribution of trees and rocky lands, farmer ability to pay, farmer behavior, and preference for certain operations and cropping patterns. This data are then analyzed and used to make decisions while deploying machinery. Not only are these data very useful for planning deployment of machines, they also are very useful for planning and deciding on what field support services that would be necessary for team deployed. Such is found to have a positive contribution to efficiency and productivity of tractors and operators. Between 2017 and 2018, the business has recorded a 300% growth in productivity – expanding its operations from single service to six integrated services. The focus has also now changed from trying to offer single operations on 45,000 acres a year in multiple locations to only 15,000 acres for a combination of a least three service operations.
Conclusion
The case of AgroMech Limited is possible for any company. Actionable information from the growing pile of data can lead to better business decision making that can then increase productivity and revenue, and manage costs and growth. Though singular, this case proves that data can make business decision making, even in a complicated and confusing market as mechanization, clearer and easier. AgroMech Limited no longer has to worry about bank statements as their only measure for growth and health of the business. As market systems development practitioners, we intend to use this learning to encourage more businesses to adopt a similar approach. The era of using gut feelings or drawing lots to make business decisions are over. Whether in a thin market or not, every business must position themselves to harness the power of data in order to have better chance of survival and growth. For market systems practitioners, this is the next frontier for supporting systemic change and subsequently transforming thin markets.
[1] MIT Sloan School of Business
[2]Rachkara and Olido (2017) Economic Viability for Agricultural Mechanization Business in Northern Uganda. GUJ http://www.guj.ac.ug
Comments